“Legislation is lagging behind” is something that we often hear when it comes to the field of artificial intelligence. This is because on the one hand, this field continues to advance rapidly, making all sorts of interesting applications possible. But on the other hand, not everything that’s technically possible should be done.
To balance the use of AI within its borders, the EU recently adopted its Artificial Intelligence Act (AIA) to bring more clarity to what can actually be done with AI, and what needs to be considered if AI is applied in a so-called “high risk” context.
Even though it will take 2 years after AIA’s entry into force for the legislation to become fully applicable, knowing how to implement it and be compliant with it is already relevant. This is because some of the AIA’s requirements will be applicable sooner, and others can already help companies minimize the risk of ending up with an irresponsible application of AI. Refer to the timeline below.
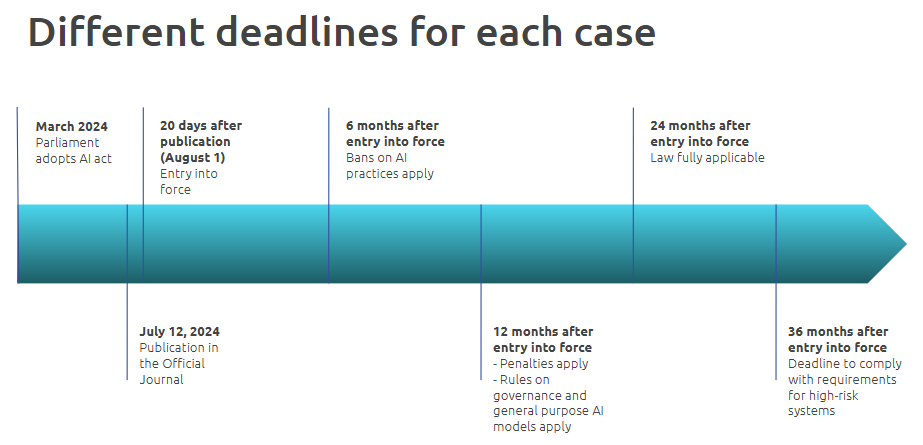
While there are various perspectives for this new legislation, this blog focuses on exploring what needs to be technically fixed to make AI that is categorized as high-risk comply with the AIA.
What is the Artificial Intelligence Act (AIA) and what does it do?
In 2021, the European Commission proposed a new EU regulatory framework on artificial intelligence: the Artificial Intelligence Act (AIA). It is the first law on AI by a major regulator worldwide. As of March 2024, the final text was adopted by the EU parliament. The first prohibitions will apply from February 2025, 6 months after the Act's entry into force on the 1st of August 2024.
This regulation has the twin objective of promoting the uptake of AI on the one hand, and of addressing the risks associated with certain uses of this technology on the other. It aims to give people the confidence to embrace AI-based solutions while ensuring that the fundamental rights of European citizens are protected.
It is good to point out that the AIA is intended only to capture the commercial placing and usage of AI products in the EU. It excludes AI systems used solely for the purpose of scientific research; law enforcement; military, defense and national security; as well as systems developed in the course of a purely personal, non-professional activity.
What is AI according to the EU?
The AIA impacts applications of AI, specifically software products that can influence the environments that they interact with. The legislation considers that a key characteristic of AI systems is their capability to infer, that transcends basic data processing, enabling learning, reasoning or modeling. This spans a suite of software development frameworks that encompass:
- Machine learning
- Logic and knowledge based approaches
Therefore, almost any software decision support system operating within the EU qualifies as AI. This means that those systems are not only limited to machine learning systems, but also expand to cover expert rule-based systems. Moreover, not only systems that are made in the EU itself are subject to the legislation; any provider or distributor of AI services that reach the EU market also needs to be compliant.
What are the categories of AI according to the AIA?
To unravel this regulation, it is useful to get a better understanding of the classification it applies. In the AIA, there is a distinction between AI practices (i.e. the goal an AI system is used for), AI systems (which are classified using a risk approach), and general-purpose AI models (which are developed without an specific end goal).
A substantial number of applications would qualify as high-risk applications, such as many applications in the financial sector or HR-related AI systems. And even if an application is qualified as having limited or minimal risk, the EU still encourages the developers and users to comply with the rules as set for the high-risk applications.
The AIA applies the following AI systems classification:
Prohibited AI practices:
These AI applications should be abandoned because they cause too much risk for health, safety or fundamental rights of European citizens, e.g. AI applications that are used towards social scoring by public authorities or private entities.
Risk approach for AI systems:
AI systems are divided in two risk categories:
- High-risk systems: These include systems developed within some specific areas, such as recruitment, immigration, biometric identification, critical infrastructure, credit worthiness evaluation, amongst others. A system is also considered high risk if it is used as a safety component of a product falling under the EU health and safety laws (like medical devices or toys), and systems used to conduct conformity assessments on other AI systems. ‘High-risk’ AI systems will have to comply with a strict set of requirements for trustworthy AI. These systems are only permitted on the EU market if they have been subjected to (and successfully withstood) a conformity assessment, and must be registered in an EU database.
- Other systems: These are mostly subject to transparency obligations. This category includes (but is not limited to) systems that interact with humans directly, emotion recognition and biometric classification systems, or systems that generate synthetic audio, image, video or text content. Similar obligations to GDPR apply for this category: Users should be notified when they are interacting with such systems, and should be informed of the operation of the system. For content generation systems, it is required to disclose whether the content was generated or manipulated by AI. Of course, other laws such as GDPR are still applicable, so all personal data should be processed according to relevant law.
General-purpose AI models
The regulation places certain obligations on the providers of these models. In particular, technical documentation and documentation for third parties who intend to integrate the model should be made available and kept up to date. This also applies to compliance with other relevant regulation (copyright law) and creating and making a publicly available, detailed summary of the data used for training.
Within this category, models can be classified as having systemic risk if they require a high computational power during training, or equivalent high capabilities such a high number of model parameters, high input and output modalities, or high number of end users. For these models, additional obligations apply, such as monitoring, documenting and reporting potential incidents, and assessing risks at Union level, amongst others.
Achieve your organization's full potential with the leading AI consultancy in the Netherlands
How does the AIA promote AI innovation?
The goal of the EU’s AIA is to create a legal framework that is innovation-friendly, future-proof and resilient to disruption. Therefore, apart from all the requirements and obligations mentioned, the AIA contains measures that support innovation. In particular, it requires competent national authorities to set up AI regulatory sandboxes within 24 months of the entry of the regulation into force. This establish a controlled environment to test innovative technologies for a limited time on the basis of a testing plan.
Even though The Netherlands is yet to gain experience in the domain of AI regulatory sandboxes, they have been already tested in countries such as Norway and the UK. This sandbox regulation is an appropriate complement to a strict liability approach, given the need to maintain a balance between a protective regulation on the one hand, and innovation in the rapidly developing AI field on the other*.
What are the necessary technical adjustments if your AI is labeled as high-risk by the AIA?
The first thing to consider is that the AI act differentiates four entity types, depending how AI is being used, namely:
- Deployers: Refers to those in the EU using an AI system that isn’t self-developed.
- Importers: These are persons or companies using an AI system trademarked outside of the EU (e.g Github CoPilot).
- Providers: Those who develop their own models in-house and later put them on the market under their trademark.
- Distributors: Those acting as middlemen in between the developers of an AI model and the users of said model.
It is worth noting that the AIA sets specific rules for each of the above in regards to high-risk AI systems. In this blog, we will lay out the generic rules that apply to all of them. If you want to know more about the specific requirements you should comply with for your use case, feel free to get in touch with one of Xomnia’s AI governance experts.
We will use AI systems that perform credit scoring as an example to answer the question above. Credit scoring is a statistical analysis performed by a machine learning algorithm to predict someone’s creditworthiness. It is used, for instance, by lenders and financial institutions to determine the creditworthiness of a person or a small, owner-operated business.
The AIA clearly prohibits the practice of ‘social scoring’ in which an AI system assigns or increases and decreases individual scores based on certain behavior (for surveillance purposes). This is because credit scoring comes with the risk of using information that is not relevant in determining the credit score, increasing the chances of discrimination and bias (e.g. using ethnic background to determine if one could apply for a loan).
For instance, a study conducted at UC Berkeley found that fintech algorithms are less biased than face-to-face lenders, but there are still some challenges. According to the study, even though fintech algorithms charge minority borrowers 40% less on average compared to what face-to-face lenders would charge, they still assign extra mortgage interest to borrowers from African or Latin American backgrounds.
However, the AIA classifies using financial information to predict creditworthiness as high-risk AI, allowing it only when it complies to the following requirements:
1. Creating and maintaining risk management systems: It should be possible to identify, estimate and evaluate potential risks related to such systems, e.g. the risk of erroneous or biased AI-assisted decisions due to bias in the historical data. This should be planned as a continuous and iterative process over the whole lifecycle of the system.
For example, due to a confounding interaction with lower salaries being associated with certain zip codes, a model can offer lower limits to credit applications from zip codes where ethnic minorities tend to live. This may happen even though the original model did not have race or ethnicity as an input to check against. [source: Harvard business review].
Therefore, removing bias from a credit decision requires more adjustment than simply removing data variables that clearly suggest gender or ethnicity. Biases in historical data should be identified and rectified to provide an artificial, but more accurate credit assessment. For example, a possibility to adjust for this latent bias is to apply different thresholds to different groups to balance out the effects in the historical data. Toolkits like IBM AI Fairness 360 or Fairlearn contain tools to detect and compensate for imbalances between groups and individuals.
2. Testing: Testing should be carried out and include real-world conditions to ensure that high-risk systems are compliant with the first point, or in other words, to identify the most appropriate and targeted risk management measures. It can be done at any point but in all cases, this should be before the system is placed on the market or put into service.
For example, when training risk scoring algorithms, it is important to test the trained models on datasets that represent real life, including individuals from different backgrounds and social groups. It is advisable to perform testing in early stages of development to identify latent bias or other risks and create appropriate measures. Specific requirements apply to the datasets used for training, validating and testing, as we elaborate in the following point.
3. Applying adequate data governance: Governance practices should be conducted, in addition to ensuring the quality of the datasets used. This includes implementing governance practices concerning data collection, carrying out relevant data preparation (e.g. annotation), formulating assumptions and examining possible biases in data. Furthermore, training, validating and testing datasets should be relevant, representative, free of errors and complete when it comes to the intended purpose of the system. Manual interventions to detect and correct for unfairness like that might result in a more equitable credit system.
Additionally, datasets should take into account specific geographical, contextual, behavioral or functional conditions in which the system will be used. This can be achieved by collecting data specific to the geography where the risk scoring will be performed, but might also include manual adjustments to appropriately reflect other conditions.
General examples of how this can be done are scaling of input data, data transformations, data enrichments and enhancements, manual and implicit binning, sample balancing and creeping (new) data biases. Personal data may be processed, but only when subject to appropriate safeguards.
4. Writing and keeping up to date a single set of technical documentation: This set of documentation must demonstrate that the system complies with all the requirements for high-risk systems in a clear and comprehensive way. It shall contain at minimum:
- A general description of the system, including (but not limited to) its intended purpose, software versions, and instructions of use for the deployer.
- A detailed description of the elements of the system (such as description of the system architecture, design specifications, training methodologies, etc.).
- Detailed information about the monitoring, function and control of the system.
- Description of the appropriateness of the performance metrics.
- Detailed description of the risk management system in place.
- Description of relevant changes made through the lifecycle of the system.
- When applicable, a list of the harmonized EU standards applied.
- A copy of the EU declaration of conformity. Specific requirements apply to this declaration, for example, it must include the name and address of the provider (or, when applicable, their authorized representative).
- A detailed description of the system used to evaluate performance after the high-risk AI system has been put on the market.
As can be seen, the conditions for the technical documentation are rather thorough. Thus, taking sufficient time and involving AI governance experts when writing the technical documentation can be determining factors in ensuring compliance.
5. Keeping an automatic record (‘logs’): Systems should enable logging so that it is possible to identify situations that present a risk, perform post-market monitoring, and allow deployers to monitor the operation of the system to identify potential risks. There are specific requirements in place for remote biometric identification systems, which we will not elaborate on in this blog as credit scoring systems don’t typically include these systems.
Establishing a logging system early on before the release of the high-risk system into the market is a crucial step when working with high-risk AI systems.
Join the leading data & AI consultancy in the Netherlands. Click here to view our vacancies
6. Guaranteeing the transparency and traceability of the AI system: Provide all the necessary information (including instructions of use) to deployers to assess the compliance of the systems with the requirements set for a high-risk AI system.
To enable the exercise of important procedural fundamental rights, such as the right to an effective remedy, a fair trial, the right of defense and the presumption of innocence, AI systems should be sufficiently transparent, explainable and documented. Therefore, complete and detailed documentation on the process, including system architecture, algorithmic design, model specifications, as well as the intended purpose of the AI system, should be created and maintained. For the traceability, this also means proper version control of models and (train/test) data should be in place.
7. Keeping human oversight within AI systems: Human owners should be able to fully understand the capacities, limitations and output of the AI system to be able to detect and address irregularities as soon as possible. This brings in the importance of the automatic recording of events (‘logs’) while AI is operating to ensure traceability, as well as including a user interface so that the system can be effectively overseen by humans. Human users should be also able to intervene in an AI system by either disregarding its outcomes through a ‘stop button’, or by overriding or reversing its output.
8. Designing and developing high-risk AI systems to achieve appropriate levels of accuracy, robustness and cybersecurity: The level of accuracy and metrics used to determine it should be communicated to the users of high-risk AI systems. Moreover, the AI system should be resilient in regards to errors, faults or inconsistencies that may occur, and perform consistently throughout its lifecycle. This should include potential attempts by unauthorized third parties to alter its use, outputs or performance. Any technical vulnerabilities of the system should be identified and addressed to avoid attacks such as data poisoning or model poisoning.
In the case of credit scoring, an algorithm could be regularized by including an extra parameter that penalizes the model if it starts treating subclasses differently, which can contribute to its robustness. Moreover, mitigation measures for potential feedback loops should be put in place.
Conclusion
The lack of attention to data and AI ethics exposes companies to reputational, regulatory and legal risks. Therefore, the biggest tech companies have put together fast-growing teams to tackle the ethical problems that arise from AI. The AIA, being the first attempt worldwide at regulating the use of AI, brings an unprecedented regulatory challenge to the EU. Early movers could have a real competitive advantage on top of doing their moral duty.
To create future-proof AI systems, the AIA should be studied closely, as it impacts use of AI systems significantly. These systems carry potential as well as risks, such as discrimination, exploiting vulnerability, among others. Fortunately, the AIA enforces good practices when it comes to mitigating such risks. It makes creating trustworthy AI a socially responsible pursuit, clarifies what Responsible AI is, and levels the playing field by enforcing these standards across the EU.
* Truby, J., Brown, R., Ibrahim, I., & Parellada, O. (2021). A Sandbox Approach to Regulating High-Risk Artificial Intelligence Applications. European Journal of Risk Regulation, 1-29. doi:10.1017/err.2021.52